– Europe/Lisbon
Room P3.10, Mathematics Building — Online
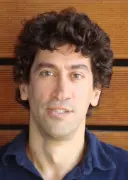
Rui Castro, Mathematics Department, TU Eindhoven
Anomaly detection for a large number of streams: a permutation/rank-based higher criticism approach
Anomaly detection when observing a large number of data streams is essential in a variety of applications, ranging from epidemiological studies to monitoring of complex systems. High-dimensional scenarios are usually tackled with scan-statistics and related methods, requiring stringent distributional assumptions for proper test calibration. In this talk we take a non-parametric stance, and introduce two variants of the higher criticism test that do not require knowledge of the null distribution for proper calibration. In the first variant we calibrate the test by permutation, while in the second variant we use a rank-based approach. Both methodologies result in exact tests in finite samples. Our permutation methodology is applicable when observations within null streams are independent and identically distributed, and we show this methodology is asymptotically optimal in the wide class of exponential models. Our rank-based methodology is more flexible, and only requires observations within null streams to be independent. We provide an asymptotic characterization of the power of the test in terms of the probability of mis-ranking null observations, showing that the asymptotic power loss (relative to an oracle test) is minimal for many common models. As the proposed statistics do not rely on asymptotic approximations, they typically perform better than popular variants of higher criticism relying on such approximations. Finally, we demonstrate the use of these methodologies when monitoring the content uniformity of an active ingredient for a batch-produced drug product, and monitoring the daily number of COVID-19 cases in the Netherlands.
Based on joint work with Ivo Stoepker, Ery Arias-Castro and Edwin van de den Heuvel:
https://arxiv.org/abs/2009.03117