– Europe/Lisbon
Online
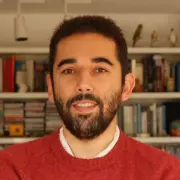
The ALeRCE astronomical alert broker
A new generation of large aperture and large field of view telescopes is allowing the exploration of large volumes of the Universe in an unprecedented fashion. In order to take advantage of these new telescopes, notably the Vera C. Rubin Observatory, a new time domain ecosystem is developing. Among the tools required are fast machine learning aided discovery and classification algorithms, interoperable tools to allow for an effective communication with the community and follow-up telescopes, and new models and tools to extract the most physical knowledge from these observations. In this talk I will review the challenges and progress of building one of these systems: the Automatic Learning for the Rapid Classification of Events (ALeRCE) astronomical alert broker. ALeRCE is an alert annotation and classification system led by an interdisciplinary and interinstitutional group of scientists from Chile since 2019. ALeRCE is focused around three scientific cases: transients, variable stars and active galactic nuclei. Thanks to its state-of-the-art machine learning models, ALeRCE has become the 3rd group to report most transient candidates to the Transient Name Server, and it is enabling new science with different astrophysical objects, e.g. AGN science. I will discuss some of the challenges associated with the problem of alert classification, including the ingestion of multiple alert streams, annotation, database management, training set building, feature computation and distributed processing, machine learning classification and visualization, or the challenges of working in large interdisciplinary teams. I will also show some results based on the real‐time ingestion and classification using the Zwicky Transient Facility (ZTF) alert stream as input, as well as some of the tools available.