– Europe/Lisbon
Online
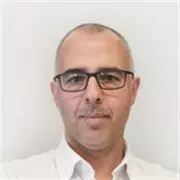
Diogo Gomes, KAUST
Mathematics for data science and AI - curriculum design, experiences, and lessons learned
In this talk, we will explore the importance of mathematical foundations for AI and data science and the design of an academic curriculum for graduate students. While traditional mathematics for AI and data science has focused on core techniques like linear algebra, basic probability, and optimization methods (e.g., gradient and stochastic gradient descent), several advanced mathematical techniques are now essential to understanding modern data science. These include ideas from the calculus of variations in spaces of random variables, functional analytic methods, ergodic theory, control theory methods in reinforcement learning, and metrics in spaces of probability measures. We will discuss the author's experience designing an applied mathematics curriculum on data science and draw on the author's experience and lessons learned in teaching an advanced course on the mathematical foundations of data science. This talk aims to promote discussion and exchange of ideas on how mathematicians can play an important role in AI and data science and better equip our students to excel in this field.