– Europe/Lisbon
Online
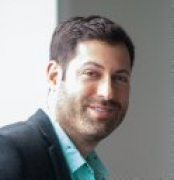
Building (and breaking) neural networks that think fast and slow
Most neural networks are built to solve simple pattern matching tasks, a process that is often known as “fast” thinking. In this talk, I’ll use adversarial methods to explore the robustness of neural networks. I’ll also discuss whether vulnerabilities of AI systems that have been observed in academic labs can pose real security threats to industrial systems. Then, I’ll present methods for constructing neural networks that exhibit “slow” thinking abilities akin to human logical reasoning. Rather than learning simple pattern matching rules, these networks have the ability to synthesize algorithmic reasoning processes and solve difficult discrete search and planning problems that cannot be solved by conventional AI systems. Interestingly, these reasoning systems naturally exhibit error correction and robustness properties that make them more difficult to break than their fast thinking counterparts.