– Europe/Lisbon
Online
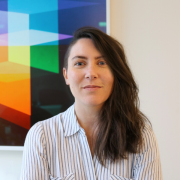
Interactive learning for Neurosciences - Between Simulation and Reality
Learning a behaviour to conduct a given task can be achieved by interacting with the environment. This is the crux of reinforcement learning (RL), where an (automated) agent learns to solve a problem through an iterative trial-and-error process. More specifically, an RL agent can interact with the environment and learn from these interactions by observing a feedback on the goal task. Therefore, these methods typically require to be able to intervene on the environment and make (possibly a very large number of) mistakes. Although this can be a limiting factor in some applications, simple RL settings, such as bandit settings, can still host a variety of problems for interactively learning behaviours. In other situations, simulation might be the key.
In this talk, we will show that RL can be used to formulate and tackle data acquisition (imaging) problems in neurosciences. We will see how bandit methods can be used to optimize super-resolution imaging by learning on real devices through an actual empirical process. We will also see how simulation can be leveraged to learn more sequential decision making strategies. These applications highlight the potential of RL to support expert users on difficult task and enable new discoveries.