– Europe/Lisbon
Online
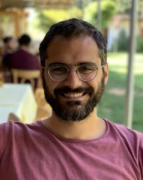
Dimensionally Consistent Learning with Buckingham Pi
Dimensional analysis is a robust technique for extracting insights and finding symmetries in physical systems, especially when the governing equations are not known. The Buckingham Pi theorem provides a procedure for finding a set of dimensionless groups from given measurements, although this set is not unique. We propose an automated approach using the symmetric and self-similar structure of available measurement data to discover the dimensionless groups that best collapse this data to a lower dimensional space according to an optimal fit. We develop three data-driven techniques that use the Buckingham Pi theorem as a constraint: (i) a constrained optimization problem with a nonparametric function, (ii) a deep learning algorithm (BuckiNet) that projects the input parameter space to a lower dimension in the first layer, and (iii) a sparse identification of nonlinear dynamics (SINDy) to discover dimensionless equations whose coefficients parameterize the dynamics. I discuss the accuracy and robustness of these methods when applied to known nonlinear systems.