– Europe/Lisbon
Online
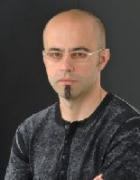
Deep neural networks, universal approximation, and geometric control
Deep neural networks have drastically changed the landscape of several engineering areas such as computer vision and natural language processing. Notwithstanding the widespread success of deep networks in these, and many other areas, it is still not well understood why deep neural networks work so well. In particular, the question of which functions can be learned by deep neural networks has remained unanswered.
In this talk we give an answer to this question for deep residual neural networks, a class of deep networks that can be interpreted as the time discretization of nonlinear control systems. We will show that the ability of these networks to memorize training data can be expressed through the control theoretic notion of controllability which can be proved using geometric control techniques. We then add an additional ingredient, monotonicity, to conclude that deep residual networks can approximate, to arbitrary accuracy with respect to the uniform norm, any continuous function on a compact subset of $n$-dimensional Euclidean space by using at most $n+1$ neurons per layer. We will conclude the talk by showing how these results pave the way for the use of deep networks in the perception pipeline of autonomous systems while providing formal (and probability free) guarantees of stability and robustness.