– Europe/Lisbon
Online
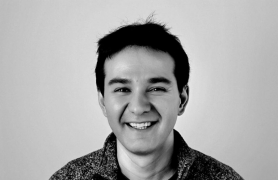
Modern Hopfield Networks in AI and Neurobiology
Modern Hopfield Networks or Dense Associative Memories are recurrent neural networks with fixed point attractor states that are described by an energy function. In contrast to conventional Hopfield Networks, their modern versions have a very large memory storage capacity, which makes them appealing tools for many problems in machine learning and cognitive and neuro-sciences. In this talk I will introduce an intuition and a mathematical formulation of this class of models, and will give examples of problems in AI that can be tackled using these new ideas. I will also explain how different individual models of this class (e.g. hierarchical memories, attention mechanism in transformers, etc.) arise from their general mathematical formulation with the Lagrangian functions.
References:
- D.Krotov, J.Hopfield, "Dense associative memory for pattern recognition"
- D.Krotov, J.Hopfield, "Large Associative Memory Problem in Neurobiology and Machine Learning"
- M.Demircigil, et al., "On a model of associative memory with huge storage capacity"
- H.Ramsauer, et al., "Hopfield Networks is All You Need"
- D.Krotov, "Hierarchical Associative Memory"