– Europe/Lisbon
Room P3.10, Mathematics Building — Online
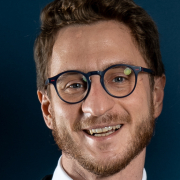
Statistical physics through the lens of real-space mutual information
Identifying the relevant coarse-grained degrees of freedom in a complex physical system is a key stage in developing effective theories. The renormalization group (RG) provides a framework for this task, but its practical execution in unfamiliar systems is fraught with ad hoc choices. Machine learning approaches, on the other hand, though promising, often lack formal interpretability: it is unclear what relation, if any, the architecture- and training-dependent learned "relevant" features bear to standard objects of physical theory.
I will present recent results addressing both issues. We develop a fast algorithm, the RSMI-NE, employing state-of-art results in machine-learning-based estimation of information-theoretic quantities to construct the optimal coarse-graining. We use it to develop a new approach to identifying the most relevant field theory operators describing a statistical system, which we validate on the example of interacting dimer model. I will also discuss formal results underlying the method: we establish equivalence between the information-theoretic notion of relevance defined in the Information Bottleneck (IB) formalism of compression theory, and the field-theoretic relevance of the RG. We show analytically that for statistical physical systems the "relevant" degrees of freedom found using IB compression indeed correspond to operators with the lowest scaling dimensions, providing a dictionary connecting two distinct theoretical toolboxes.