– Europe/Lisbon
Online
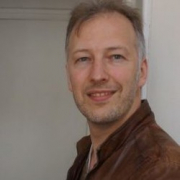
Some exactly solvable models for statistical machine learning
The increasing dimensionality of data in the modern machine learning age presents new challenges and opportunities. The high-dimensional settings allow one to use powerful asymptotic methods from probability theory and statistical physics to obtain precise characterizations and develop new algorithmic approaches. There is indeed a decades-long tradition in statistical physics with building and solving such simplified models of neural networks.
I will give examples of recent works that build on powerful methods of physics of disordered systems to analyze different problems in machine learning and neural networks, including overparameterization, kernel methods, and the gradient descent algorithm in a high dimensional non-convex setting.
Additional file
Projecto FCT UIDB/04459/2020.