– Europe/Lisbon
Online
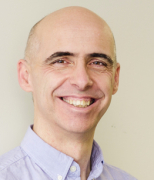
Confident Off-Policy Evaluation and Selection through Self-Normalized Importance Weighting
Off-policy evaluation is the problem of predicting the value of a policy given some batch of data. In the language of statistics, this is also called counterfactual estimation. Batch policy optimization refers to the problem of finding a good policy, again, given some logged data.
In this talk, I will consider the case of contextual bandits, give a brief (and incomplete) review of the approaches proposed in the literature and explain why this problem is difficult. Then, I will describe a new approach based on self-normalized importance weighting. In this approach, a semi-empirical Efron-Stein concentration inequality is combined with Harris' inequality to arrive at non-vacuous high-probability value lower bounds, which can then be used in a policy selection phase. On a number of synthetic and real datasets this new approach is found to be significantly superior than its main competitors, both in terms of tightness of the confidence intervals and the quality of the policies chosen.
The talk is based on joint work with Ilja Kuzborskij, Claire Vernade and Andras Gyorgy.
Additional file
Projecto FCT UIDB/04459/2020.