– Europe/Lisbon
Online
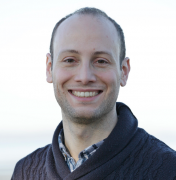
Efficient Bayesian computation by proximal Markov chain Monte Carlo: when Langevin meets Moreau
This talk summarises some new developments in Bayesian statistical methodology for performing inference in high-dimensional inverse problems with an underlying convex geometry. We pay particular attention to problems related to imaging sciences and to new stochastic computation methods that tightly combine proximal convex optimisation and Markov chain Monte Carlo sampling techniques. The new computation methods are illustrated with a range of imaging experiments, where they are used to perform uncertainty quantification analyses, automatically adjust regularisation parameters, and objectively compare alternative models in the absence of ground truth.
Additional file
Projecto FCT UIDB/04459/2020.