– Europe/Lisbon
Online
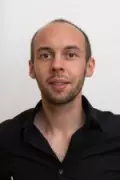
Andreas Döpp, Ludwig-Maximilians-Universität München | Faculty of Physics
Machine-learning strategies in laser-plasma physics
The field of laser-plasma physics has experienced significant advancements in the past few decades, owing to the increasing power and accessibility of high-power lasers. Initially, research in this area was limited to single-shot experiments with minimal exploration of parameters. However, recent technological advancements have enabled the collection of a wealth of data through both experimental and simulation-based approaches.
In this seminar talk, I will present a range of machine learning techniques that we have developed for applications in laser-plasma physics [1]. The first part of my talk will focus on Bayesian optimization, where I will showcase our latest findings on multi-objective and multi-fidelity optimization of laser-plasma accelerators and neural networks [2-4].
In the second part of the talk, I will discuss machine learning solutions for tackling complex inverse problems, such as image deblurring or extracting 3D information from 2D sensors [5-6]. Specifically, I will discuss various adaptations of established convolutional network architectures, such as the U-Net, as well as novel physics-informed retrieval methods like deep algorithm unrolling. These techniques have shown promising results in overcoming the challenges posed by these intricate inverse problems.
References
- Data-driven Science and Machine Learning Methods in Laser-Plasma Physics
- Expected hypervolume improvement for simultaneous multi-objective and multi-fidelity optimization
- Multi-objective and multi-fidelity Bayesian optimization of laser-plasma acceleration
- Pareto Optimization of a Laser Wakefield Accelerator
- Measuring spatio-temporal couplings using modal spatio-spectral wavefront retrieval
- Hyperspectral Compressive Wavefront Sensing