– Europe/Lisbon
Online
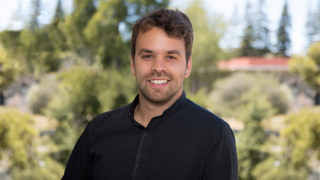
Accelerating the understanding of nonlinear dynamical systems using machine learning
The description of nonlinear, multi-scale dynamics is a common challenge in a wide range of physical systems and research fields — from weather forecast to controlled nuclear fusion. The development of reduced models that balance between accuracy and complexity is critical to advancing theoretical comprehension and enabling holistic computational descriptions of these problems. I will discuss how techniques from statistical and machine learning are offering new ways of inferring reduced physics models from the increasingly abundant data of nonlinear dynamics produced by experiments, observations, and simulations. In particular, I will focus on how sparse regression techniques can be used to infer interpretable plasma physics models (in the form of nonlinear partial differential equations) directly from the data of first-principles fully-kinetic simulations. The potential of this approach is demonstrated by recovering the fundamental hierarchy of plasma physics models based solely on particle-based simulation data of complex plasma dynamics. I will discuss how this data-driven methodology provides a promising tool to accelerate the development of reduced theoretical models of nonlinear dynamical systems and to design computationally efficient algorithms for multi-scale simulations.