– Europe/Lisbon
Online
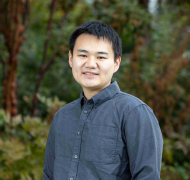
Simon Du, University of Washington
Provable Representation Learning
Representation learning has been widely used in many applications. In this talk, I will present our work, which uncovers when and why representation learning provably improves the sample efficiency, from a statistical learning point of view. I will show
- the existence of a good representation among all tasks, and
- the diversity of tasks are key conditions that permit improved statistical efficiency via multi-task representation learning.
These conditions provably improve the sample efficiency for functions with certain complexity measures as the representation. If time permits, I will also talk about leveraging the theoretical insights to improve practical performance.