– Europe/Lisbon
Online
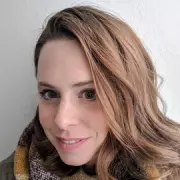
Sara Magliacane, University of Amsterdam and MIT-IBM Watson AI Lab
Causal vs causality-inspired representation learning
Causal representation learning (CRL) aims at learning causal factors and their causal relations from high-dimensional observations, e.g. images. In general, this is an ill-posed problem, but under certain assumptions or with the help of additional information or interventions, we are able to guarantee that the representations we learn are corresponding to some true underlying causal factors up to some equivalence class.
In this talk I will first present CITRIS, a variational autoencoder framework for causal representation learning from temporal sequences of images, in systems in which we can perform interventions. CITRIS exploits temporality and observing intervention targets to identify scalar and multidimensional causal factors, such as 3D rotation angles. In experiments on 3D rendered image sequences, CITRIS outperforms previous methods on recovering the underlying causal variables. Moreover, using pretrained autoencoders, CITRIS can even generalize to unseen instantiations of causal factors.
While CRL is an exciting and promising new field of research, the assumptions required by CITRIS and other current CRL methods can be difficult to satisfy in many settings. Moreover, in many practical cases learning representations that are not guaranteed to be fully causal, but exploit some ideas from causality, can still be extremely useful. As examples, I will describe some of our work on exploiting these "causality-inspired" representations for adapting policies across domains in RL and to nonstationary environments, and how learning a factored graphical representations (even if not necessarily causal) can be beneficial in these (and possibly other) settings.